Paper
View all working papersFeatured Posts
View all postsFeatured Press Posts
View all press posts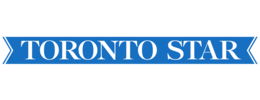
Adopted by more than 200 cities in the United States, 311 service-request systems are one of the most significant links between residents and city government, accounting for more than eight million requests annually in New York City alone. Increasingly, these data are being used to develop predictive models of resident concerns and problem conditions across a city. However, predictive models trained on these data can suffer from biases embedded in the propensity to complain (or make a request) that can vary based on socio-economic and demographic characteristics of an individual or neighborhood, cultural differences that can affect residents’ willingness to interact with their government, and widely divergent local conditions. The goal of this project is to analyze the factors that influence resident reporting and to develop models to account for individual and neighborhood differences in reporting behaviors. Objectives include the identification and measurement of algorithmic bias in city management and the development of more fair, transparent methods for improved public-sector resource allocation.
Please fill out the information below to receive our e-newsletter(s).
*Indicates required.