Related
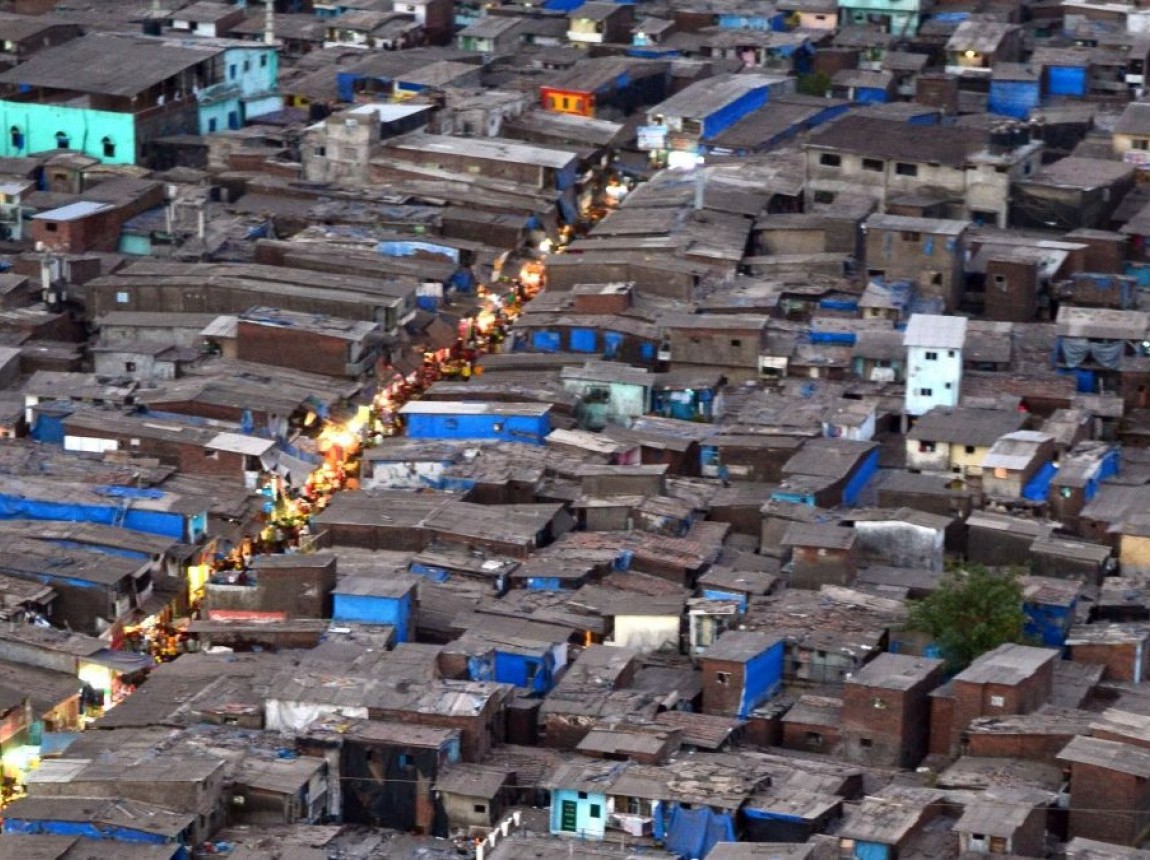
Nov 24,2021
This article was written by Shlomo Angel, Patrick Lamson-Hall, Alejandro M. Blei, Eric Mackres and Brookie Guzder-Williams
Rationale:
Intra-urban land use maps provide important information to urban planners and policymakers, but these maps are costly, time consuming to generate and are often unavailable in developing countries where most urban growth is now occurring. This paper reports on machine learning methods to automate the production of land use maps from cloud-free mosaics of Sentinel-2 imagery. We have trained a novel neural network architecture to produced 5 meter resolution land use maps for a global stratified sample of 200 cities. The sample includes all world regions, 78 countries, and a range of population sizes. The model architecture is roughly 1 to 2 orders of magnitude smaller than similar architectures such as UNet (Ronneberger, Fischer, & Brox, 2015) and DeeplabV3+ (Chen, Zhu, Papandreou, Schroff, & Adam, 2018), significantly lowering the cost and computational requirements to produce maps. We are in the process of generating land use maps for all 4,000 + cities and metropolitan areas in the world with populations exceeding 100,000. The resulting product will be the first, regularly updated, freely available, global intra-urban land use maps at 5 meter resolution.
We present a 4-tier land use taxonomy which at its root distinguishes open-space from built-up area. At the second tier, it subdivides the built-up category into nonresidential and residential areas. The third tier distinguishes formal from informal residential land use, and the fourth tier further subdivides formal and informal residential land uses into more detailed categories. Accuracy scores at tier-1 and tier-2 were 86% and 79% respectively. Tiers 3 and 4 had an accuracy scores of 75% and 71% respectively. Additionally, we train a roads-only model and compare its output to the Atlas of Urban Expansion’s Arterial Roads dataset and Open Street Map. As an example use case, we train an Informal Settlement Classifier, correctly classifying 87% of the settlements.
Please fill out the information below to receive our e-newsletter(s).
*Indicates required.